Overview
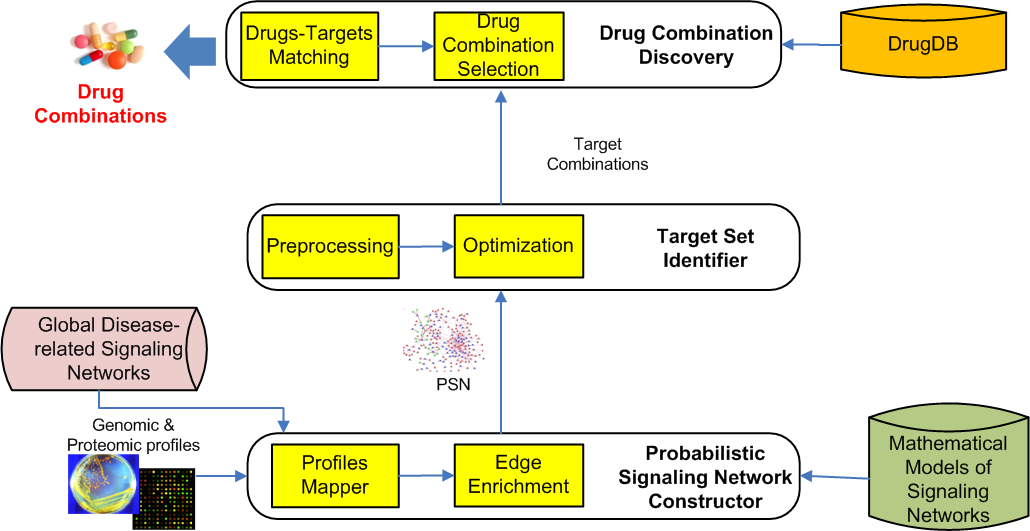
Combination therapy, where several drugs interact with multiple targets, holds tremendous promise for effective clinical outcomes in the management of chronic, complex diseases. In this research, we take a step towards this grand goal by laying out the vision of a novel in silico, data-driven combination therapy research for complex network diseases. Given the genomic and proteomic profiles of patients (e.g., cancer patient), the precarious goal is to automatically predicts “optimal” set of synergistic drug combinations and corresponding dosages, which can potentially achieve the therapeutic goal while minimizing any off-target effects. Specifically, we explore how to construct a disease-related (cancer-related) signaling network by integrating publicly-available disease-specific signalling networks with omics data. Next, topology and dynamics of the network are analyzed as a whole using novel network analytics techniques to identify promising target combinations with desirable properties (e.g., synergistic in nature, good efficacy and minimum off-target effect) to regulate the activities of key disease-related molecular players. Finally, optimal candidate drug combinations to modulate these targets are predicted by integrating and analyzing drug information with the target nodes.
Through this research, we seek to validate the hypotheses that disease-specific signaling network can be created from publicly-available biological networks and expression profiles; topological and dynamic features of signaling networks when combined with these omics data can reveal critical information of potential drug targets in the network; and integration of publicly available drug-related information can enable in silico inference of superior drug combinations for the predicted target combinations. A salient feature of our research is that it does not await the availability of complete models of disease-related signaling networks that realistically capture, in mathematical form, actual cellular signaling events. Instead, in our research we aim to predict superior target/drug combinations by accepting the noisiness and incompleteness of the network.
Successful realization of this research can result in an effective platform for in silico screening of drug combinations in a rational way, by aiding early discovery of suitable combination therapy and guiding the design of further in vitro and in vivo experiments. Consequently, this will not only lead to significant cost savings in clinical trials for drugs but also pave the way for discovering superior drug combinations to battle complex diseases. Results of this research are published in venues such as Bioinformatics journal, ACM BCB, and Methods. This research is partially funded by NTU Tier 1 Complexity Grant, AcRF MOE Tier 2 Grant, and SMA-CSB Grant.
Key Contributions
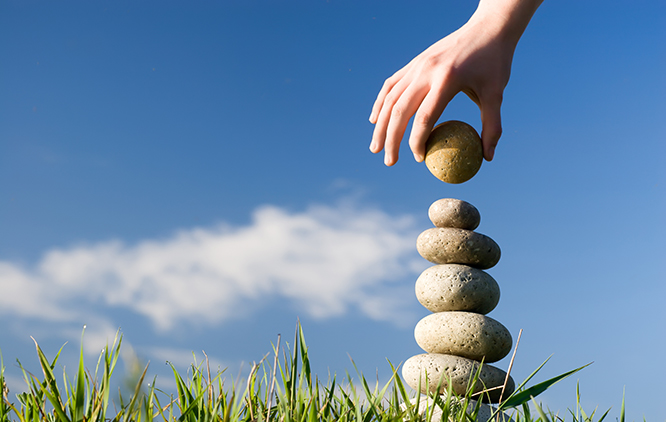
- TROVE (Bioinformatics 2018) is the world's first platform to facilitate analysis and visualization of hallmark-based human cancer signaling network.
- TENET (Bioinformatics 2015, ICDM 2016) is world's first technique to automatically characterize known targets in a signaling network
- World's first in silico framework called MASCOT (Methods 2017) that predicts target combinations in a signaling network that are synergistic and off-target effect-aware.
Current Members
Past Members
- Nerissa XU
- Huey Eng CHUA
- Qing ZHAO
Collaborators
- C Forbes DEWEY, Jr
MIT - Lisa TUCKER-KELLOGG,
Duke-NUS - Hanry YU
NUS - Jie ZHENG
Shanghai Inst. of Tech. - Jiancheng HU
National Cancer Centre, Singapore
Software
- TROVE
Facilitates hallmark annotation, visualization and analysis in cancer signaling networks. - TINTIN
A network-based approach that ranks a given set of networks based on its similarity to a reference network. - TAPESTRY
A network-based approach that prioritizes candidate targets in a given signaling network. - TENET
Characterizes known targets in signaling networks using topological features.